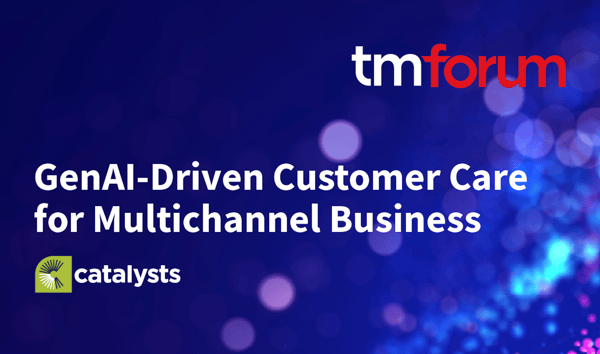
Tupl Inc. to showcase AI-Powered customer care solution at Innovate Asia 2024
2024-10-14 · Press Releases
By now, we have long past the point of having to explain the business value of AI and ML; the key right now is how to properly implement a machine learning project and successfully push it to production. MLOps tries to do exactly that: automating and productizing machine learning applications and workflows.
However, it remains true that Machine-learning (ML) models almost always require deployment to a production environment to provide business value. The reality, though, is that many models never make it to production, and if they do, the deployment process takes much longer than necessary. Why? Before deciding on an MLOps strategy and tool, there are several aspects to consider, and all too often, some of them are overlooked.
The market is booming with MLOps platforms with different functionalities, so it can be a daunting task to decide which one to implement if we are not aware of those fundamental aspects we need to look for in an MLOps platform. In this article we analyze the most important underlying principles to consider when selecting a suitable ML platform, in particular for addressing automation use cases in the Telecom industry.
Tupl has been developing ML applications and taking them to production for quite some time now, and we have found there are three key basic features an MLOps platform needs to have to ensure successful deployment of a ML model to production:
1. Flexibility and Scalability.
One of the most important aspects of a best-in-class MLOps platform is its flexibility, which should allow an organization to respond quickly to any requirements' changes, and to retrain the model based on new data sets if needed. To achieve this flexibility, it is important for an MLOps platform to be an open framework that allows to develop and adapt solutions to the ongoing and evolving needs quickly. Also, ML models require monitoring data quality and the automation of model retraining in an easy way. It is also important to make sure the platform is scalable and fit to develop new use cases that might arise. One of the keys to achieving this is making sure the MLOps platform of choice can be managed easily without necessarily requiring a data scientist profile to do so: this reduces the risks associated to transferring models from the data science team to the subject matter experts' team and vice versa. Having a platform that requires very little coding ensures that flexibility and scalability, as well as ensuring simplified maintenance.
2. Fast Time-to-Market.
Let’s not forget ML models are software and deploying and maintaining any software is a complex task, to which ML introduces added complexities. Having the right MLOps tool streamlines the process of developing and deploying ML models, reducing project delivery time and costs. Once in production, ML applications need to be monitored for invalid predictions or data drifts. A key point when talking about time-to-market is the ability to provide an MLOps platform in a SaaS form. With this approach it is easier and quicker to get started, including a faster procurement process, which allows to have a functional solution in operation within weeks.
3. Available Use Cases.
Each industry has specific use cases that have specific requirements, data sources to integrate, etc. An ideal MLOps platform will have already built models that address the most common needs in that particular industry.
Deloitte summarizes these features that define an optimal MLOps platform in telco very well in this quote: “ML platforms dedicated to telecoms are another crucial component in RAN automation. Such platforms show traits of low code and should be designed to be used by engineers with basic knowledge of data science and analytics, as opposed to generic ML frameworks which are designed for data scientists. - Deloitte, “The Age of Telecom Network Automation”.
Communication Service providers (CSP) are one of the key businesses where automation can make a huge difference, from improving customer service operations through automatic resolution of technical issues to saving thousands of dollars in energy consumption thanks for automated power saving features.
TuplOS is an MLOps platform that was first implemented in the Telco industry, though it has already been successfully used in other industries such as Smart Agriculture or Smart Manufacturing.
TuplOS® platform provides a simple, flexible, and scalable framework to create end-to-end automation applications relying on a series of UI-based utilities that facilitate transferring ideas into real products with no programming required, as it is designed for domain experts.
TuplOS is based on the pillars that work as differentiators for any industry and that are already making a difference in many Telco operators around the world: time to market, flexibility, scalability, and industry-specific use cases for streamlined and more efficient ML model deployment.
TuplOS allows data teams to achieve faster model development, delivery, and deployment to production of accurate ML models.
For example, to develop a solution from scratch that implements a centralized NOC for an autonomous car fleet it takes 900+ man-days for a team of 9 employees without TuplOS. In comparison, less than 75 man-days and 4 employee profiles are needed to design a similar solution with TuplOS. Projects are scaled faster; months are sliced to days.
As an example, below is the detailed calculation of time required to deploy a project from scratch with and without TuplOS. However, the calculation mentioned in this table is flat and includes only the cost of a project setup; but it doesn’t take into account the cost of solution acquisition, negotiations, and procurement which are the essential components of Time to Market definition. Based on our calculations of the delivered use cases – market research, shortlist and procurement of the project takes up to ¼ of the overall project budget.
Picture “Decomposition of the resources needed to deploy the project from scratch with and without TuplOS”
A key advantage of the TuplOS platform is that it has many machine learning models already developed for Telco Use Cases. For years, Tupl has been working with Telco operators to address the most important use cases, so the platform has been shaped to adjust to those particular needs, which differentiates it from many other platforms available.
Here is a sample of some of the use cases Tupl has developed for Telco Operations Automation:
- Network Optimization: network service quality control and predictive maintenance.
Picture: Network Advisor Use Cases, built on TuplOS
- Customer Engagement: real-time interaction and proactive customer needs prediction to provide personalized engagement to increase telecom vendor’s brand loyalty and reduce customer churn.
Picture: AI Care Use Cases, built on TuplOS
- Advanced Visualization: customized for telco-purposes analytics views and widgets to create and display the required relevant information for analysis and reporting.
Picture: “TuplOS® Advanced UI Framework – Unifier”
- RAN Energy Saving: Operators can achieve significant energy savings while maintaining a positive customer experience leveraging the TuplOS platform to utilize near real-time data of the user experience and network traffic, resulting in a 10% reduction of their electricity bill.
There are multiple other use cases already deployed, and most importantly, the Tupl platform has the flexibility to quickly build any other automation use case. As a matter of fact, in our article The Real Business Value of Automating Operations with TuplOS®, we develop in depth several use cases in Telco and how using the right platform can lead to a much faster time-to-market with reduced costs.
Platforms need to be flexible and scalable to offer immediate value to a customer. A platform with a modular architecture and collaborative environment provides organizations with the necessary flexibility to get up and running quickly by enabling each of the team members to run the platform's functionality with no dependencies.
TuplOS MLOps platform is designed as fully open and customizable by the customer, offering easy plug-and-play components so that each user can make decisions on the best cloud, database, repositories, and other components to use. Modular architecture enables teams to leverage their entire suite of tools and seamlessly bring specific components of the platform into their ML workflows.
TuplOS non-code Machine Learning platform allows domain experts to digitalize their knowledge into mathematical models without requiring a deep knowledge of statistical analysis or machine learning techniques.
TuplOS is a collaborative environment that facilitates real-time collaboration for experiment tracking, feature engineering, model management, and controlled model transitioning, deployment, and monitoring performed from the web interface or even mobile phone. It allows to setup of a new cluster within minutes, manage multiple clusters simultaneously, choose data integration configuration, cloud, or on-premises storage – all to create ML models seamlessly and in a flexible way.
MLOps platforms need to enable vast scalability and management where thousands of models can be controlled, managed, and monitored for continuous integration, continuous delivery, and deployment. Particularly, they need to be scalable to address changing requirements in a project, or additional data sources to be taken into consideration.
TuplOS has easy data ingestion and management process to ensure the applications are scalable to respond to any change in requirements.
It is the time for Telcos to achieve fast and reliable hyper-automation that not only preserves the customer experience but actually improves it. In order to achieve that, it is mandatory to rely on an MLOps platform that is flexible, scalable, fast and that allows to immediately automate the most used use cases in the Telco industry.
TuplOS Platform is an MLOps platform that provides the integration, intelligence, flexibility, and control needed to deliver a comprehensive solution no matter what the current digitalization level is. It leverages industry-leading artificial intelligence and machine learning capabilities to provide end-to-end synchronized delivery of the new applications in the fastest and most cost-effective way.
“Tupl MLOps Platform for Telecommunications enables telecom companies to develop, deploy and manage AI applications at scale, transforming AI projects into real-world business outcomes. With Tupl, you can build and run AI models in real-time, deploy them anywhere (cloud or on-prem), and bring to life your most ambitious data-driven strategies for smoother and safer communications.” - Pablo Tapia, CTO & Co-founder at Tupl
Get a demo of TuplOS today: https://www.tupl.com/tuplos/
2024-10-14 · Press Releases
2024-10-02 · Press Releases
2024-06-06 · Press Releases · Others
Get started and request a demo to learn how our solutions can help you.