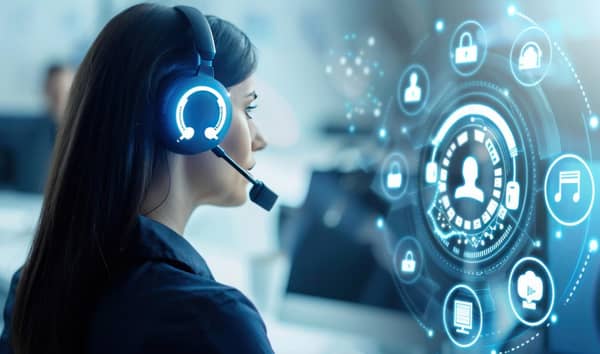
How AI Is Revolutionizing First Call Resolution (FCR) in Customer Service?
2025-02-07
The Telco network infrastructure faces unprecedented operational challenges and complexities: new technologies and services combined with an increasing number of users, devices, and the associated quality of service requirements. In 2022, the proliferation of networked devices reached 28.5 billion, underscoring the critical need for efficient network management and troubleshooting in Telco operations. This burgeoning scale, coupled with the demand for digital transformation— forecasted to reach 3.9 trillion U.S. dollars globally in 2027 according to Statista - highlights the necessity for Telco operators to adopt AI network troubleshooting automation and leverage technologies such as machine learning (ML), deep learning (DL), and natural language processing (NLP) to enhance productivity and address the escalating OpEx spent on troubleshooting, which amounts to USD 60 billion annually. (Source: Cisco)
The digital transformation of operations, transitioning from manual effort for network troubleshooting to AI-driven solutions, not only addresses the current challenges of network complexity but also marks a significant shift towards proactive network management. As the industry gravitates towards multi-cloud usage, the integration of AI in network operations emerges as a pivotal strategy for Telcos. This evolution from manual to automated processes, underscored by AI represents a leap towards optimizing network quality and minimizing downtime, thereby empowering Telco operators to navigate the intricate landscape of modern network troubleshooting with enhanced efficiency and precision.
Efficient network troubleshooting is of utmost importance for Telco operators. It is crucial for them to be able to quickly identify and resolve any network issues that may arise or ideally even before they occur. The main reason is that network issues can lead to downtime, which can be highly detrimental to their operations. By promptly addressing these issues, Telco operators can minimize any potential disruptions, ensure the best possible customer experience, and maximize the network performance.
By adopting AI-driven solutions and leveraging advanced technologies, Telco operators can improve customer satisfaction. Efficient network troubleshooting ensures that any network issues are resolved promptly, minimizing any potential downtime that customers may experience. This leads to a more reliable and stable network, which in turn enhances the overall customer experience.
To achieve efficient network troubleshooting, Telco operators can adopt AI-driven solutions, which can analyze vast amounts of network data and identify patterns or anomalies that may indicate potential issues. This allows operators to proactively address these issues before they escalate and cause significant disruptions.
Furthermore, AI-driven solutions can streamline network management processes. They can automate routine tasks, such as network monitoring and performance analysis, freeing up valuable time for network engineers to focus on more critical tasks. This automation not only improves operational efficiency but also reduces the risk of human error, which can sometimes occur during manual network management processes.
Manual network troubleshooting presents several limitations that can significantly hinder the efficiency and effectiveness of addressing network issues:
Additionally, manual methods are plagued by several operational challenges:
1. Overwhelmed by Data: In business contexts, the sheer volume of data can be overwhelming, making manual troubleshooting impractical.
2. Prone to Human Error: The risk of human error is high, which can lead to inaccurate troubleshooting results and extended resolution times.
3. Reactive Nature: Traditional manual troubleshooting is reactive, focusing on fixing issues after they occur rather than preventing them, which is less efficient in maintaining network health.
These limitations underscore the need for more sophisticated and automated solutions in network troubleshooting to enhance efficiency, accuracy, and proactive problem resolution.
AI plays a crucial role in simplifying network troubleshooting by automating various tasks and providing proactive insights:
- Scalability: AI allows operations and engineering teams to manage larger network infrastructures without proportionally increasing the manual effort required. This scalability is essential as networks grow in complexity and size.
- Shift in Focus: By automating routine data analysis tasks, AI frees up operations and engineering professionals to concentrate on strategic, high-value activities, thus enhancing overall productivity and innovation within the organization.
- Example: AI-driven systems can detect a sudden drop in network performance and immediately correlate it with a recent configuration change or an external network event, leading to faster resolution.. Focus on what matters: AI can help prioritize the detected network issues and ensure that the most customer-impacting problems are handled with priority.
- Automated Solutions: AI and machine learning technologies automate various aspects of the network lifecycle, including design, configuration, and optimization. This automation not only reduces the manual workload but also increases the accuracy and speed of network troubleshooting and maintenance.
- Continuous Improvement and Proactivity: Through self-learning and optimization mechanisms, AI systems continuously monitor, evaluate, and improve network performance, ensuring that the network adapts to changing demands and remains efficient over time.
These advancements underscore the transformative impact of AI on network troubleshooting, shifting the paradigm from manual, reactive measures to proactive, AI-driven solutions.
AI-driven network operations are transforming the landscape of telecommunications, offering numerous benefits that significantly improve efficiency and customer satisfaction. Here are the key advantages:
1. Predictive Analytics for Network Health: AI tools analyze historical data to predict potential network failures, enabling preemptive action to avoid downtime.
2. Smart Resource Allocation: Dynamic resource allocation powered by AI ensures optimal network performance by adjusting resources according to real-time needs and priorities.
3. Routine Task Automation: From configuration management to performance optimization, AI automates routine tasks, minimizing human errors and operational costs.
1. Intelligent Solutions: AI enhances customer experience by predicting user behavior and minimizing network disruptions, ensuring seamless connectivity.
2. AI-Powered Chatbots: Immediate, intelligent responses to customer inquiries via AI chatbots enhance service quality and customer satisfaction.
These benefits underscore the transformative impact of AI in telecommunications, driving enhanced operational efficiency and superior customer experiences.
Tupl’s Network Advisor is an AI-powered solution that simplifies network troubleshooting for telecom operators. It integrates with your existing network data sources, including configurations, topology, and performance metrics. The AI engine then analyzes this data to automatically detect performance issues, pinpoint root causes, and even recommend corrective actions. This frees up engineers’ time and allows them to focus on more strategic tasks. Network Advisor’s flexibility means it can adapt to your specific network environment and tackle any relevant performance issue. Overall, it fosters a collaborative approach between AI and human expertise to streamline troubleshooting and optimize network operations. Here's a closer look at some key use cases demonstrating the capabilities of Tupl Network Advisor:
One of the biggest challenges for telecom operators is delivering a consistently optimal user experience, which is achieved and measured with a key metric called user data speed (or throughput). However, radio cells can sometimes underperform, leading to sluggish data transfer rates and frustrating user experiences.
Tupl Network Advisor addresses this challenge head-on with its Low User Throughput use case. Leveraging AI and automation, our solution tackles this issue in several ways:
The benefits of addressing low user throughput are clear. By swiftly identifying and resolving these issues, Tupl Network Advisor helps ensure a seamless user experience for your customers. This translates into increased user satisfaction, reduced troubleshooting times that minimize disruptions, and ultimately, a more optimized network that delivers on its full potential.
Ensuring seamless network coverage is paramount for a positive user experience. However, occasionally radio cells can become dormant, meaning they cease handling user traffic optimally. This can lead to dropped calls, slow data speeds, and frustrated customers.
Tupl Network Advisor's Sleeping Cells Anomaly Detection use case tackles this challenge head-on through automation and AI. Here's how it works:
The benefits of proactively addressing sleeping cells are clear. Tupl Network Advisor's AI-powered approach minimizes user impact by detecting and rectifying anomalies before they cause widespread disruptions. This translates to operational efficiency for your team and a more reliable network for your customers.
RTWP Anomaly Detection is a critical component of mobile network management. Its primary purpose is to identify and classify anomalies related to "Received Total Wideband Power" (RTWP), which directly impact network performance and customer experience. These anomalies can arise from various root causes, including:
1. Ongoing Site Construction: When new cell sites are being deployed or existing ones are under construction, RTWP levels may deviate from the norm.
2. New Band Deployment: Introducing new frequency bands can lead to RTWP fluctuations.
3. Ongoing Network Tickets: Network maintenance activities or troubleshooting tickets can affect RTWP.
4. RAN Equipment Alarms: Alarms triggered by radio access network (RAN) equipment may impact RTWP.
5. Antenna Imbalance Due to Connectors: Poorly connected antennas can cause RTWP irregularities.
6. External Interference or PIM (Passive Intermodulation): Interference from neighboring networks or faulty equipment can distort RTWP.
7. Hardware Issues: Faulty components within the network infrastructure can lead to RTWP anomalies.
The solution leverages AI models to correlate data from diverse sources, such as performance and configuration data, performance logs, network alarms, etc. By analyzing this information, it identifies abnormal RTWP patterns and recommends appropriate actions. Whether adjusting antenna parameters, scheduling maintenance, or addressing hardware issues, the goal is to optimize network performance and enhance user experience.
Like other Network Advisor use cases, this solution's flexibility allows it to adapt to various scenarios, making it a valuable asset in maintaining a robust mobile network in a constantly changing environment.
After analyzing the performance of deployed use cases, we can confirm the following business impact points:
The telecom industry is on the brink of a transformative leap, driven by the rapid advancement and integration of AI technologies. This shift is not merely theoretical but is backed by substantial financial growth and strategic planning:
Source 1 Precedence Research
These insights paint a vivid picture of a future where AI is not just an auxiliary tool but the cornerstone of telecommunications, driving innovation, efficiency, and service quality to unprecedented levels.
Conclusion
The transformative journey from manual to AI-driven network troubleshooting outlines a trajectory toward more efficient, reliable, and proactive telecommunications operations. By embracing AI and machine learning, Telcos are now positioned to navigate the complexities of modern network infrastructure with precision and agility. This evolution not only streamlines operational processes but also significantly enhances the customer experience by reducing downtime and improving service quality. Tupl Network Advisor exemplifies the power of AI in action. This solution empowers Telco operators to address critical network challenges, ensuring a superior user experience and optimized network performance.
As the digital landscape continues to evolve, the role of AI in telecommunications becomes increasingly pivotal. Tupl Network Advisor stands as a testament to the substantial advantages of adopting AI automation for Telcos. This strategic shift not only fortifies networks against present and future challenges but also sets the groundwork for unprecedented levels of efficiency and customer satisfaction in the telecom industry. By integrating AI-driven solutions, Telcos can confidently navigate the path towards a future of smarter, more efficient, and customer-centric network operations.